Freshwater Explorer
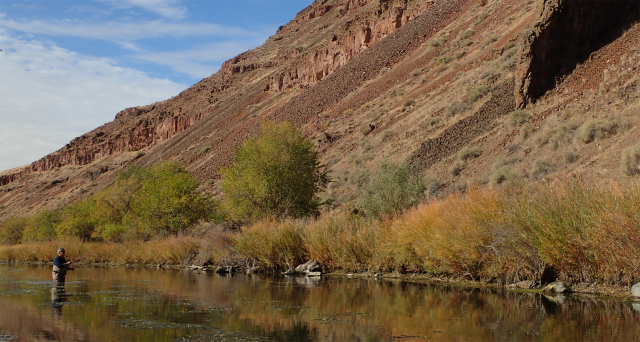
On This Page:
- Compatibility and Availability
- Capabilities
- Applications
- Quick Guides
- Background Information
- Related Resources
- Technical Support
- Citations
- Datasets and R-code
Access Freshwater Explorer (Version 1.0)
Access Freshwater Explorer (Version 2.0)
A tool for exploring the water quality of freshwater resources
EPA's Freshwater Explorer is an interactive web-based mapping tool for water quality parameters of freshwater streams, lakes, and groundwater wells in all 50 U.S. states, Puerto Rico, and the U.S. Virgin Islands. It includes both observed data points and estimated background concentrations to make it easy to identify areas where water quality has been impacted by human activities. It can be used by citizens and non-governmental organizations, to better understand national and local water quality issues and to provide water quality information to help federal, state, territory, Tribal, and local partners make decisions about freshwater resources. Users can add spatial layers to explore associations between water quality measurements and natural and human geographical factors and any of the more than 10,000 other available data layers accessible from the GeoPlatform that may affect water quality in the United States.
Disclaimer: Any mention of trade names, manufacturers, or products does not imply an endorsement by EPA. EPA and its employees do not endorse commercial products, services, or enterprises.
Compatibility and Availability
The Freshwater Explorer is map-centric and mobile-friendly. It works on all screen sizes, from desktop computers and tablets to mobile phones. The application can be downloaded to a third-party PC with full access to modify it with an ArcGIS account.
Preferred citation: Wharton C., Olson J., Newcomer-Johnson, T., Cormier S. 2025. U.S. EPA Freshwater Explore V2. United States Environmental Protection Agency. April 2025. https://arcg.is/Xfneu
Capabilities
The original release of EPA’s Freshwater Explorer Version 1.0 allows users to quickly access and visualize predicted background salt and mineral levels for approximately 2.65 million stream segments of a few kilometers each.
Freshwater Explorer Version 2.0 expands that capability to 12 commonly sampled water quality parameters. Users can conduct geographical searches, access information about how maps and water quality values are estimated in Freshwater Explorer, and access datasets and published papers. The tool includes summary statistics for observed levels for approximately 289,000 stream locations, 10,000 lakes, and 50,000 groundwater wells. New water quality parameters are forthcoming, so check back regularly. Users can use basic views or perform complex searches. Users can also modify a customizable map and pull in data from other EPA sources such as ECHO (Enforcement Compliance History Online) and ATTAINS (Assessment Total Maximum Daily Load [TMDL] Tracking and Implementation System).
Please Note: Freshwater Explorer 1.0 is scheduled for decommissioning in August 2025. Freshwater Explorer 2.0 will remain online in its place, offering enhanced functionality for users.
Exchange Portal
EPA’s Freshwater Explorer 2.0 uses data stored in EPA’s Water Quality Exchange (WQX) through the Water Quality Portal (WQP) and makes those data more accessible and intuitive to use. The Freshwater Explorer curates and summarizes the Portal’s hundreds of millions of water quality data records from more than 500 federal, state, Tribal, and other partners. The tool was developed to help users visualize water quality datasets with color-coded displays. EPA compiled and curated data from the WQP using Tools for Automated Data Analysis (TADA). EPA curated data from the WQX, removing some and tagging very low or high values submitted by contributors. However, data still include undetected errors of magnitude and location, and therefore, the tool is most appropriate for performing screening-level analysis. Users should assess the suitability for their specific applications.
Data and Metadata
EPA’s Freshwater Explorer 1.0 and 2.0 provide unique data and pull data from multiple databases from federal agencies through web services. Below is a summary of data included in Freshwater Explorer. Freshwater Explorer 2.0 provides the same information as version 1.0 with additional data layers and capabilities.
EPA’s Freshwater Explorer 1.0 and 2.0 both contain:
- Predicted background conductivity: Predicted values of natural background conductivity from empirical models from EPA’s Water Quality Portal (WQP).
- Model Validation: Shows the predictive performance of the natural Predicted Background Stream Conductivity model at reference sites that were used for model development, and the metadata provides details on model development.
- Observed Conductivity - WQP: Observational data from EPA’s Water Quality Portal (WQP), the nation's largest source for water quality monitoring data, cooperatively sponsored by the United States Geological Survey (USGS), the Environmental Protection Agency (EPA), and the National Water Quality Monitoring Council (NWQMC).
- Observed Conductivity - NWIS: Observational data from the U.S. Geological Survey (USGS) National Water Information System (NWIS).
- ECHO Data/System: Location and basic information from Enforcement and Compliance History Online (ECHO).
- ATTAINS Data/System: Highlights impaired streams and basic information about water quality assessments and total maximum daily loads (TMDLs), through data submitted by states under Clean Water Act sections 303(d) and 305(b).
EPA’s Freshwater Explorer 2.0 also contains:
- Estimated background conductivity: 25th centile of monitoring data from EPA's National Resource Survey Assessment (NRSA) empirical water quality parameters.
- alkalinity, ammonia, calcium, chlorophyll A, chloride, conductivity, organic carbon, pH, sulfate, total phosphorus, total nitrogen.
- Observed water quality parameters - WQP: Observational data from EPA’s Water Quality Portal (WQP), the nation's largest source for water quality monitoring data, cooperatively sponsored by the United States Geological Survey (USGS), the Environmental Protection Agency (EPA), and the National Water Quality Monitoring Council (NWQMC).
- alkalinity, ammonia, calcium, chlorophyll A, chloride, conductivity, organic carbon, pH, sulfate, total phosphorus, total nitrogen.
The most basic use of the EPA’s Freshwater Explorer is to check whether observed levels are greater than background or nearby locations. Furthermore, users can look for associations between observed water quality and land cover to begin to understand why levels may be higher than expected. From there visualizations can vary depending on the interest of the user. For example, users may choose to compare point sources with estimated background and relationships among other water quality parameters (e.g., nutrients, chlorophyll-a), potential and actual sources, (e.g., land cover and toxic release records) and demographics (e.g., population density). This combination of information can be useful for states working with communities and regulated entities to find the right balance of protection and use of freshwater resources. Background is estimated and may need to be calibrated for local conditions.
U.S. EPA Freshwater Explorer - Quick Guides
- Freshwater Explorer 1.0 - Quick Guide
Freshwater Explorer 1.0 is simpler and designed primarily for conductivity.
Please Note: Freshwater Explorer 1.0 will be retired in August 2025. Freshwater Explorer 2.0 will remain online in its place, offering enhanced functionality for users. - Freshwater Explorer 2.0 - Quick Guide
Freshwater Explorer 2.0 requires users to select water quality parameters and makes it easier to import and export data. - List of Abbreviations
- Glossary
Background Information
What is Fresh Water?
Fresh water is characterized by the concentration of dissolved mineral ions. It is essential for drinking water, agriculture, industry, and aquatic life in streams, rivers, and inland lakes. Increases in mineral and ion levels sometimes indicate a source of pollution in freshwater systems. Higher levels of minerals like phosphorus in the water can cause harmful algal blooms and affect aquatic wildlife. These conditions can increase costs for making water suitable for drinking by people and livestock, for use in agricultural and industrial processes, and for water reuse.
Ions carry a positive or negative charge which facilitates the flow of electricity in water. The more ions, the easier electricity flows between electrodes, giving scientists a way to measure the total concentration of ions. Individual kinds of dissolved ions are usually observed with ion selective probes. Total dissolved mineral and nutrient ions are usually reported as conductivity in micro Siemens per centimeter (µS/cm) or mg/L. Within the Freshwater Explorer, conductivity refers to specific conductivity (SC) in µS/cm calibrated at 25°C.
Predicted Background Conductivity
Predicted monthly average background conductivity (2000-2015) as it would occur if water quality was minimally influenced by anthropogenic sources is based on geophysical and other data at thousands of sites in the United States that were judged to be relatively pristine (Olson and Cormier 2019). The predictor variables were generated for each stream line within the National Hydrography Dataset Plus version 2 (NHDPlusV2) with a random forest model that used predictor variables accessed from StreamCat Dataset (ESRI 2012, Hill et al 2016).
For more detail see the predictive background conductivity mode model metadata page.
The Natural Background Stream Conductivity Model predicts background specific conductivity (SC) for stream segments in the contiguous United States to enable comparison with measured in-stream conductivity (Olson and Cormier 2019). The predictive algorithm uses geology, soil, vegetation, climate and other empirically measured predictors. It was developed for streams with natural background SC greater than 2000 µS/cm. Above this level, inland water is considered brackish and the Natural Background Model estimates may be less reliable.
Data for some parameters that affect background SC were not readily available and were not included in the model. These include freshwater and marine interfaces, natural mineral springs, salt deposits that may affect groundwater and streams, and other natural sources of salts. In such areas the model is likely to underestimate SC. Local knowledge is necessary when assessing differences between predicted and measured background SC. The model is less precise with intermittent flows and very arid climates.
Estimated Background for other Water Quality Parameters
The National Aquatic Resource Surveys (NARS) use statistical surveys with each sampling site representing a specific portion of the total resource or population of waterbodies, such as a proportion of all lakes in the nation or a subpopulation of only large lakes. In Freshwater Explorer 2.0, the 25th centile of a type of waterbody, e.g., lake, stream reach, wetland, etc., is calculated as an empirical background estimate. However, centile-based levels do not necessarily characterize natural background (Stoddard et al. 2006; Herlihy and Sifneos, 2008). Using this approach has inherent uncertainty because 25% of an ecoregion may be altered by human activity. Furthermore, as time passes, there is the potential for an upward creep of perceived ambient background, a shifting baseline (Pauly 1995; Soga and Gaston 2018). If ecosystems have degraded over time, thresholds set at fixed centiles progressively increase, contributing to a different perceived background and poorer water quality over time.
Because areal scale is also important, the estimated background in the Freshwater Explorer 2.0 uses the smallest geographical area with a sufficient sample size to support estimation. Nevertheless, localized ambient and natural background for naturally occurring substances may occur at greater or lesser levels than the regional or national norms and does not replace site recognizance or local knowledge.
Related Resources
-
Olson, J.R. and Cormier, S.M. 2019. Modeling Spatial and Temporal Variation in Natural Background Specific Conductivity. Environmental Science & Technology. DOI: 10.1021/acs.est.8b06777.
-
Hill, R.A., Weber, M.H., Leibowitz, S.G., Olsen, A.R. and Thornbrugh, D.J., 2016. The Stream‐Catchment (StreamCat) Dataset: A database of watershed metrics for the conterminous United States. JAWRA Journal of the American Water Resources Association, 52(1), pp.120-128.
Relevant Citations
Coffey, D.B., Cormier, S.M., Harwood, J. 2014. Using field-based species sensitivity distributions to infer multiple causes. Human and Ecological Risk Assessment: An International Journal. 20(2): 402-432.
Cormier S.M., Suter II, GW. 2013. A method for deriving water-quality benchmarks using field data. Env. Tox. Chem.2(2): 255–262.
Cormier, S.M., 2017. Data for: Field-based methods for evaluating the annual maximum specific conductivity tolerated by freshwater invertebrates. 10.23719/1371704.
Cormier, S.M., 2017. Dataset for: A Field-based Model of the Relationship Between Extirpation of Salt-intolerant Benthic Invertebrates and Background Conductivity. https://doi.org/10.23719/1371707. https://www.ncbi.nlm.nih.gov/pmc/articles/PMC7106560/
Cormier, S.M., Suter II, G.W II. A method for assessing causation of field exposure–response relationships. Env. Tox. Chem.32(2): 272–276.
Cormier, S.M., Suter II, G.W. 2013. Editorial. Sources of data for water quality criteria. Env. Tox. Chem. 32(2): 254.
Cormier, S.M., Suter II, G.W., Zheng L. 2013. Derivation of a benchmark for freshwater ionic strength Env. Tox. Chem.2(2): 263–271.
Cormier, S.M., Suter II, GW, Zheng L., Pond G. J. 2013. Assessing causation of the extirpation of stream macroinvertebrates by a mixture of ions. Env. Tox. Chem.32(2): 277–287.
Cormier, S.M., Suter, G.W., Fernandez, M.B. and Zheng, L., 2020. Adequacy of sample size for estimating a value from field observational data. Ecotoxicology and Environmental Safety, 203, p.110992
Cormier, S.M., Wilkes S.P., Zheng L. 2013. Relationship of land use and elevated ionic strength in Appalachian watersheds. Env. Tox. Chem. 32(2): 296–303.
Cormier, S.M., Zheng, L. and Flaherty, C.M., 2018. A field-based model of the relationship between extirpation of salt-intolerant benthic invertebrates and background conductivity. Science of The Total Environment, 633, pp.1629-1636.doi: 10.1016/j.scitotenv.2018.02.044.
Cormier, S.M., Zheng, L. and Flaherty, C.M., 2018. Field-based method for evaluating the annual maximum specific conductivity tolerated by freshwater invertebrates. Science of the Total Environment, 633, pp.1637-1646. doi: 10.1016/j.scitotenv.2018.01.136.
Cormier, S.M., Zheng, L., Hayslip, G. and Flaherty, C.M., 2018. A field-based characterization of conductivity in areas of minimal alteration: A case example in the Cascades of northwestern United States. Science of The Total Environment, 633, pp.1657-1666. https://doi.org/10.1016/j.scitotenv.2018.02.018.
Cormier, S.M., Zheng, L., Hill, R.A., Novak, R.M. and Flaherty, C.M., 2018. A flow-chart for developing water quality criteria from two field-based methods. Science of The Total Environment, 633, pp.1647-1656. doi: 10.1016/j.scitotenv.2018.01.137.
Cormier, S.M., Zheng, L., Leppo, E.W. and Hamilton, A., 2018. Step‐by‐step calculation and spreadsheet tools for predicting stressor levels that extirpate genera and species. Integrated Environmental Assessment and Management, 14(2), pp.174-180.
Cormier, S.M., Zheng, L., Suter, G.W. and Flaherty, C.M., 2018. Assessing background levels of specific conductivity using weight of evidence. Science of The Total Environment, 628, pp.1637-1649. doi: 10.1016/j.scitotenv.2018.02.017.
ESRI, 2014. ArcGIS Desktop: Release 10.2.2. Environmental Systems Research Institute, Redlands, California.
Griffith, M.B., Zheng, L. and Cormier, S.M., 2018. Using extirpation to evaluate ionic tolerance of freshwater fish. Environmental toxicology and chemistry, 37(3), pp.871-883.
Herlihy, A.T. and J.C. Sifneos. 2008. Developing Nutrient Criteria and Classification Schemes for Wadeable Streams in the Conterminous US. North American Benthological Society 27(4):932-948.
Hill, R.A., Weber, M.H., Leibowitz, M.H., Olsen, A.R., Thornbrugh, D.J., 2016. The Stream-Catchment (StreamCat) Dataset: A Database of Watershed Metrics for the Conterminous United States. JAWRA Journal of the American Water Resources Association, 52(1), 120-128. doi: https://doi.org/10.1111/1752-1688.12372
McKay, L., Bondelid, T., Dewald, T., Johnston, J., Moore, R., Rea, A., 2012. NHDPlus Version 2: User Guide. National Operational Hydrologic Remote Sensing Center, Washington, DC. Available at: https://nctc.fws.gov/courses/references/tutorials/geospatial/CSP7306/Readings/NHDPlusV2_User_Guide.pdf
NASA, 2019. Moderate Resolution Imaging Spectroradiometer (MODIS) satellite data. Available at: https://modis.gsfc.nasa.gov/data/
Olson, J.R. and Cormier, S.M. 2019. Modeling Spatial and Temporal Variation in Natural Background Specific Conductivity. Environmental Science & Technology. DOI: 10.1021/acs.est.8b06777. https://www.ncbi.nlm.nih.gov/pmc/articles/PMC7153567/
Olson, J.R. and Cormier, S.M., 2019. Modeling spatial and temporal variation in natural background specific conductivity: Data sets and R-code. doi: https://doi.org/010.23719/1500945
Olson, J.R., Hawkins, C.P., 2012. Predicting natural base‐flow stream water chemistry in the western United States. Water Resources Research, 48(2). doi: https://doi.org/10.1029/2011WR011088
Omernik, J. M.; Griffith, G. E., 2014. Ecoregions of the conterminous United States: evolution of a hierarchical spatial framework. Environmental. Management. 54 (6), 1249−1266. doi: https://doi.org/10.1007/s00267-014-0364-1
Pauly D. 1995. Anecdotes and the shifting baseline syndrome of fisheries. Trends Ecol Evol 10: 430.
Soga M and Gaston KJ. 2018. Shifting baseline syndrome: causes, consequences, and implications. Front Ecol Environ 16: 222–30
Stoddard, J.L., Larsen, D.P., Hawkins, C.P., Johnson, R.K. and Norris, R.H., 2006. Setting expectations for the ecological condition of streams: the concept of reference condition. Ecological applications, 16(4), pp.1267-1276. https://doi.org/10.1890/1051-0761(2006)016[1267:SEFTEC]2.0.CO;2
Suter II G.W., Cormier, S.M. 2013. A method for assessing the potential for confounding applied to ionic strength in central Appalachian streams. Env. Tox. Chem.32(2): 288–295.
U.S. Environmental Protection Agency (U.S. EPA), 2016. STORET. Available at: https://www.epa.gov/storet/
U.S. Geological Survey (USGS), 2016. National Water Information System. Available at: https://waterdata.usgs.gov/nwis
Datasets and R-code
Cormier, S.M., 2017. Data for Assessing Background Levels of Specific Conductivity Using Weight of Evidence 508 Compliant. (dataset). https://doi.org/10.23719/1402418.
Cormier, S.M., 2017. Distribution Link for Data for: A Field-based Characterization of Conductivity in Areas of Minimal Alteration: A Case Example in the Cascades of Northwestern United States. https://doi.org/10.23719/1396168.
Cormier, S.M., 2017. Data for: Estimation of field-based benchmarks from background specific conductivity. https://doi.org/10.23719/1371706
Griffith, M.B., Zheng, L. and Cormier, S.M., 2018. Data for: Using extirpation to evaluate ionic tolerance of freshwater fish. Environmental toxicology and chemistry. https://pubmed.ncbi.nlm.nih.gov/29091302
Cormier, S. M., 2017. Data Set for: Step-by-Step Calculation and Spreadsheet Tools for Predicting Stressor Levels that Extirpate Genera and Species. Doi: 10.23719/1371705
Cormier, S.M., 2017. https://github.com/smcormier/Biological-Extirpation-Analysis-Tools-BEAT/releases/tag/v.1.0.2.
Soga M and Gaston KJ. 2018. Shifting baseline syndrome: causes, consequences, and implications. Front Ecol Environ 16: 222–30
Pauly D. 1995. Anecdotes and the shifting baseline syndrome of fisheries. Trends Ecol Evol 10: 430.
Stoddard, J.L., Larsen, D.P., Hawkins, C.P., Johnson, R.K. and Norris, R.H., 2006. Setting expectations for the ecological condition of streams: the concept of reference condition. Ecological applications, 16(4), pp.1267-1276. https://doi.org/10.1890/1051-0761(2006)016[1267:SEFTEC]2.0.CO;2
Herlihy, A.T. and J.C. Sifneos. 2008. Developing Nutrient Criteria and Classification Schemes for Wadeable Streams in the Conterminous US. North American Benthological Society 27(4):932-948.
Olson, J.R. and Cormier, S.M. 2019. Modeling Spatial and Temporal Variation in Natural Background Specific Conductivity. Environmental Science & Technology. DOI: 10.1021/acs.est.8b06777. www.ncbi.nlm.nih.gov/pmc/articles/PMC7153567
Hill, R.A., Weber, M.H., Leibowitz, S.G., Olsen, A.R. and Thornbrugh, D.J., 2016. The Stream‐Catchment (StreamCat) Dataset: A database of watershed metrics for the conterminous United States. JAWRA Journal of the American Water Resources Association, 52(1), pp.120-128.